We know “raw data” is an oxymoron. Every time you choose to catalogue one thing and not another, use one measure and not another, categorize things one way and not others, clean data for some uses over others, these decisions align the data with some goals, values, and uses and (you guessed it) not others (O’Brien, 1993). It’s not inherently bad that all data is skewed, but it isn’t good when those skews go unnoticed and unchecked.
Most of our data politics methodologies are baked into projects:
- recording good metadata, including all the people who contribute to a project in any way;
- using Indigenous data sovereignty agreements over who gets to own, access, and control data from Indigenous lives, land, and bodies;
- refusing universalism and using categories for plastic pollution morphologies that fit the sources of pollution in a region rather than universally standard categories;
- and recording how we’re feeling in datasets when we’re processing samples as a way to keep that knowledge embodied and tied to emotional intelligence and fatigue.
In plastic pollution research, there are increasingly calls to standardize methods globally, but we’ve found that already standardized methods, like shoreline protocols for monitoring microplastics on shorelines, don’t work on rocky shores. We wrote one scientific paper showing how it doesn’t work, thereby setting a precedent (McWilliams, Liboiron, and Wiersma, 2018) and a a magazine article explaining the imperial and colonial roots of standardizing methods in science (Liboiron, 2020). We’ve also recently submitted a paper on how measures used (or not used) in a study align with or against Inuit governance efforts (forthcoming. Stay tuned). Finally, CLEAR Director Dr. Liboiron’s book, Pollution is Colonialism (2021), documents how threshold measures of pollution are part of colonial land relations.
Our favourite resources on moving from critique to integrating data politics into research are:
- Collaboratory for Indigenous Data Governance & the Data Warriors Lab
- OCAP Training
- D’Ignazio, Catherine, & Klein, Lauren F. (2020). Data feminism. MIT Press.
Citations and critiques of the politics of data whose work we build on:
- Bowker, Geoffrey C., & Star, Susan Leigh. (2000). Sorting things out: Classification and its consequences. MIT press.
- Canguilhem, Georges. (1991). The Normal and the Pathological. Cambridge: MIT Press.
- Hacking, Ian. (1990). The Taming of Chance. New York: Cambridge University Press.
- Liboiron, Max. (2020). Plastics in the Gut: A search for sand on a rocky shoreline upends colonial science. Orion (winter): 22-29.
- McWilliams, M., Liboiron, M., & Wiersma, Y. (2018). Rocky shoreline protocols miss microplastics in marine debris surveys (Fogo Island, Newfoundland and Labrador). Marine pollution bulletin, 129(2), 480-486.
- O’Brien, Mary H. (1993). Being a scientist means taking sides. BioScience, 43(10), 706-708.
- Pine, Kathleen H., & Liboiron, Max. (2015). The politics of measurement and action. In Proceedings of the 33rd Annual ACM Conference on Human Factors in Computing Systems (pp. 3147-3156).
- Porter, Theodore M. (1996). Trust in numbers: The pursuit of objectivity in science and public life. Princeton University Press.
- Rodriguez-Lonebear, Desi. (2016). Building a data revolution in Indian country. Indigenous data sovereignty: Toward an agenda, 253-272.
- Walter, Maggie, & Andersen, Chris. (2013). Indigenous statistics: A quantitative research methodology. Left Coast Press.
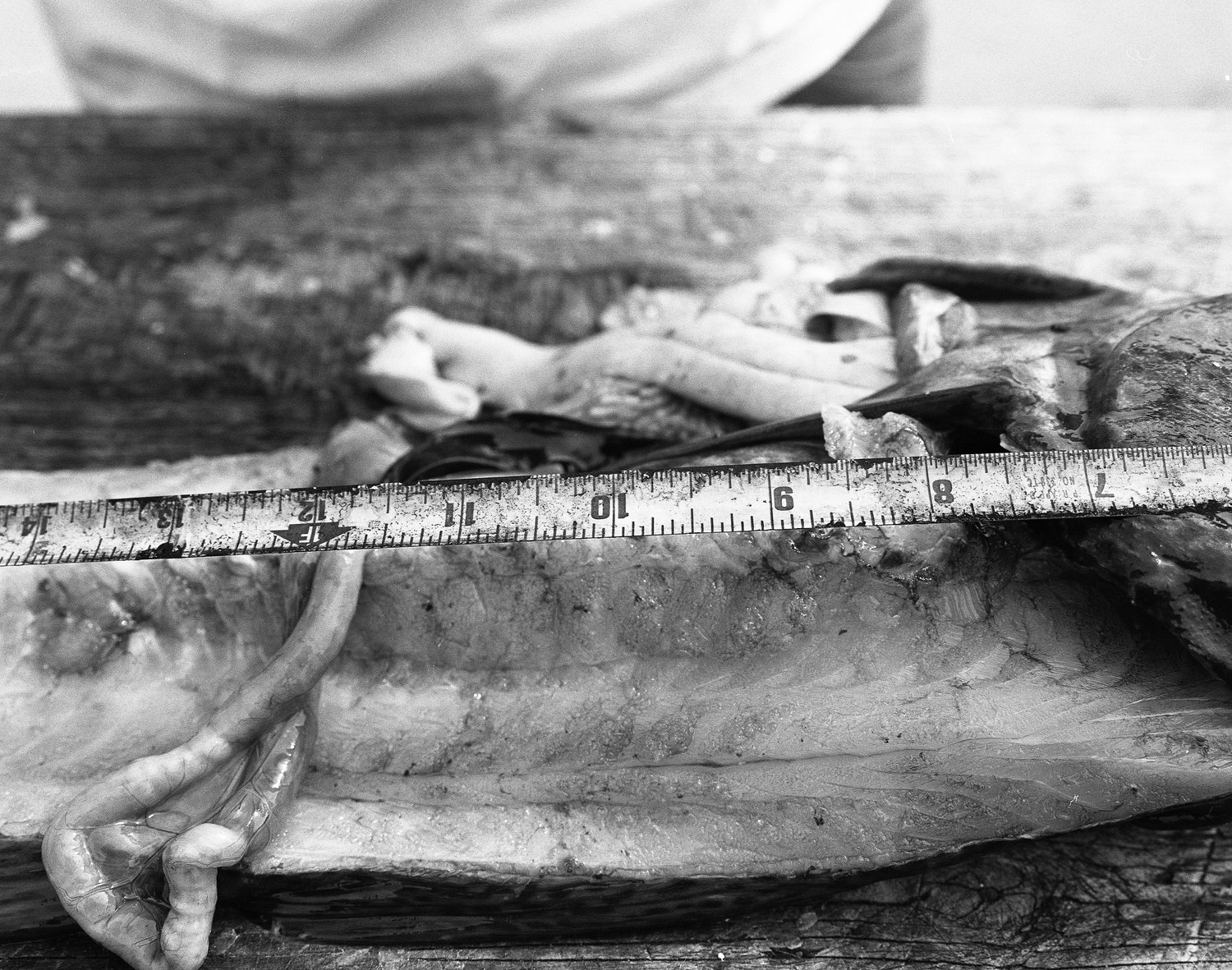